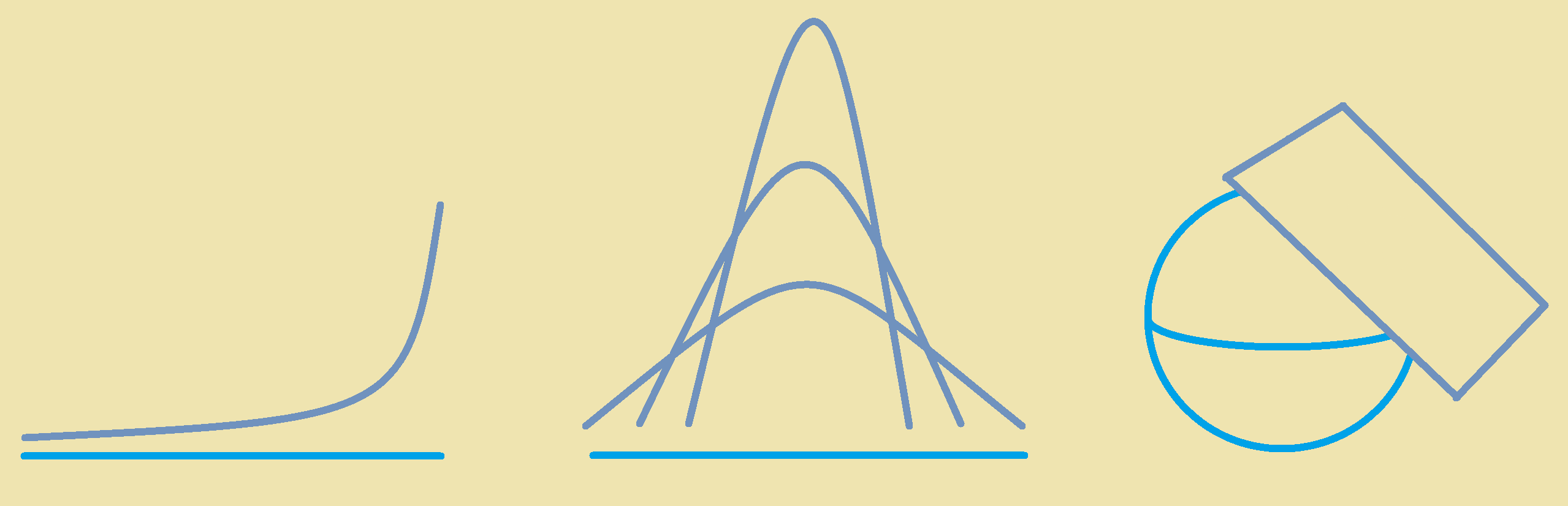
Λέσχη Μαθηματικών
Χειμερινό εξάμηνο 2024-25
26 Αίθουσα Α11 Ώρα 15:00 |
Introduction to missing data analysis Τσισμεντζόγλου Χρυσούλα Abstract: Missing data is a common issue in data analysis that occurs when some values in a dataset are absent or not recorded. The presence of missing data can significantly affect the validity and reliability of statistical analyses, leading to biased results if not properly addressed. Missing data can arise from various sources, including non-response in surveys, errors in data collection or lost information over time. Understanding the types of missingness and employing appropriate methods for handling it is crucial for ensuring robust statistical modeling and inference.In this presentation, we will explore the sources and types of missing data and discuss the fundamental methods for handling it. R code examples and their corresponding results will be highlighted throughout the presentation. Βιβλιογραφία
|
---|